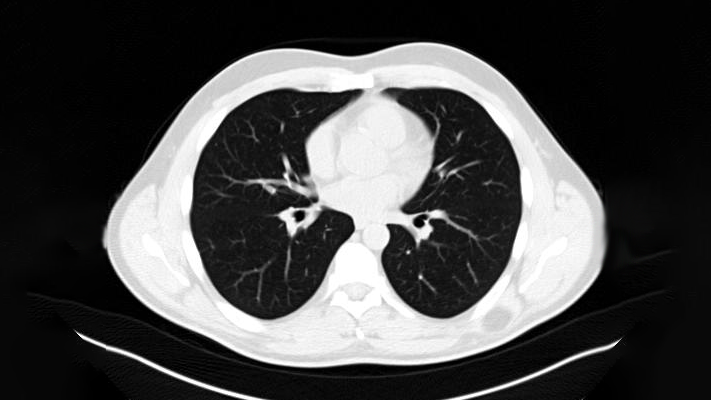
This example aim to explain how to design, train and integrate in LabVIEW environment a biomedical Unet model architecture.
Front panel overview
Diagram global overview
This section show how the model and HAIBAL functionalities are integrated inside a LabVIEW architecture design.
The architecture is composed of 3 sequential states. (Design model, Prepare data, Training model).
During the training process, we display the results in a parallel loop.
The model train process is “classic”, we repeat a sequence of Forward – Loss – Backward to process to the train of the model for each couple of inputs/outputs.
Model testΒ
Testing model consist to forward and display one featureΒ during the training (this example is not optimized, the best practice is to forward the test image after a full batch – outside of the Batch loop, we purposely did it like this to display the whole code on one page without subVI).
How to acces to this example ?
The Multi inputs outputs model train example is available in the LabVIEW find example session. Use the Keywords “Unet”Β and launch it.
The LabVIEWΒ U-Net: Convolutional Networks for Biomedical Image Segmentation is now available with the HAIBAL deep learning toolkit.
References :
https://perrine.graiphic.io/support-community/topic/using-unet-for-automatic-segmentation-of-ct-lung-images/
Thanks to Peter Herrmann from Medical Center GΓΆttingen University for providing us with the architecture of this model and the dataset of 42 images.